The Artificial Intelligence Modelling Lab (AIML) engages in a range of theoretical and applied research in Artificial Intelligence (AI) and Machine Learning (ML). Particular areas of interest include interactive theorem proving, formal modelling and verification, machine learning and its combination with higher level symbolic reasoning, as well as its application to healthcare and other complex, real-world domains.
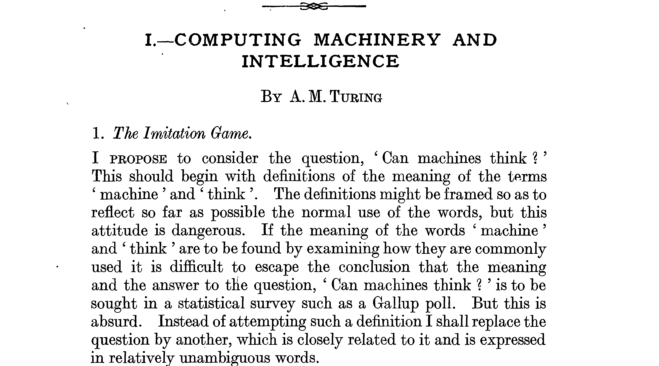
News
-
Our paper “Linear Resources in Isabelle/HOL” has just been published in the Journal of Automated Reasoning
23rd May 2024
-
Opportunity: Fully funded PhD in predicting harm from prescribed drugs
27th April 2024
-
Fiona Smith’s exhbition “The BOX” premieres at the Edinburgh Science Festival!
5th April 2024
-
Jorge Gaete Villegas passes his PhD viva
5th April 2024
-
Our second analysis of the impact of COVID-19 on Scotland’s care-homes published in Age and Ageing
17th February 2024
Some of our Existing and Past External Engagements
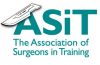
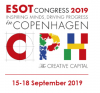
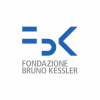
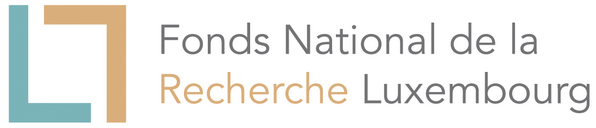
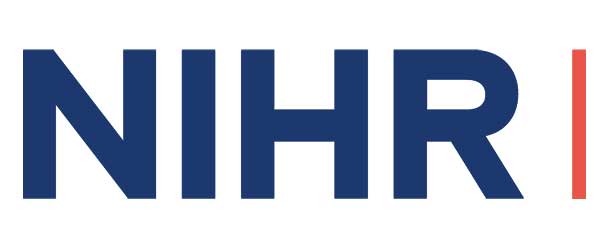
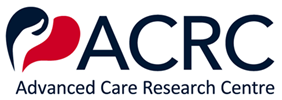
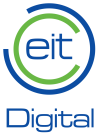
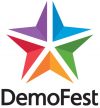
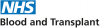
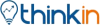
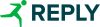
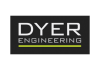
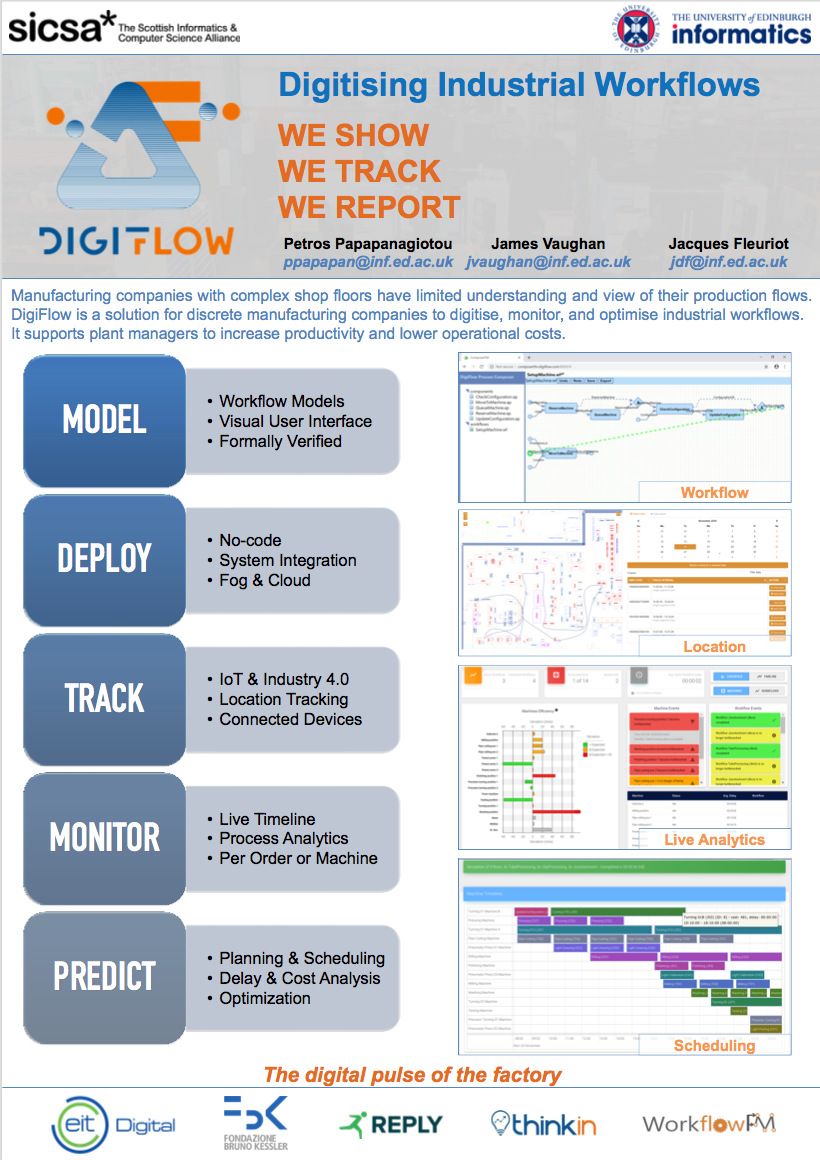
Recent Events
Predicting brain health outcomes from objectively-assessed sleep duration in UK Biobank
This presentation will presentan assessment of the feasibility of predicting brain health outcomes from sleep duration derived using accelerometery data from UK Biobank.
Speaker: Matt Whelan
Multimorbidity analysis with low condition counts: a robust Bayesian approach for small but important subgroups
In this talk, I will briefly introduce common network science approaches to multimorbidity research, present and explain our methodological approach, and present and show how our method can be easily used by the multimorbidity community via our released software package.
Speaker: Guillermo Romero Moreno
Investigating associations between physical multimorbidity and subsequent depression via a systematic cluster analysis
In this talk, we describe our investigation of associations between physical multimorbidity and subsequent depression by performing clustering analysis upon baseline morbidity data for UK Biobank participants and then performing survival analysis to compare time to subsequent depression diagnosis.
Speaker: Lauren DeLong